#Kubernetes For AI Model Deployment
Explore tagged Tumblr posts
Text
Understand how Generative AI is accelerating Kubernetes adoption, shaping industries with scalable, automated, and innovative approaches.
#AI Startups Kubernetes#Enterprise AI With Kubernetes#Generative AI#Kubernetes AI Architecture#Kubernetes For AI Model Deployment#Kubernetes For Deep Learning#Kubernetes For Machine Learning
0 notes
Text
How Is Gen AI Driving Kubernetes Demand Across Industries?
Understand how Generative AI is accelerating Kubernetes adoption, shaping industries with scalable, automated, and innovative approaches. A new breakthrough in AI, called generative AI or Gen AI, is creating incredible waves across industries and beyond. With this technology rapidly evolving there is growing pressure on the available structure to support both the deployment and scalability of…
#AI Startups Kubernetes#Enterprise AI With Kubernetes#Generative AI#Kubernetes AI Architecture#Kubernetes For AI Model Deployment#Kubernetes For Deep Learning#Kubernetes For Machine Learning
0 notes
Text
How Is Gen AI Driving Kubernetes Demand Across Industries?
Unveil how Gen AI is pushing Kubernetes to the forefront, delivering industry-specific solutions with precision and scalability.
Original Source: https://bit.ly/4cPS7G0
A new breakthrough in AI, called generative AI or Gen AI, is creating incredible waves across industries and beyond. With this technology rapidly evolving there is growing pressure on the available structure to support both the deployment and scalability of the technology. Kubernetes, an effective container orchestration platform is already indicating its ability as one of the enablers in this context. This article critically analyzes how Generative AI gives rise to the use of Kubernetes across industries with a focus of the coexistence of these two modern technological forces.
The Rise of Generative AI and Its Impact on Technology
Machine learning has grown phenomenally over the years and is now foundational in various industries including healthcare, banking, production as well as media and entertainment industries. This technology whereby an AI model is trained to write, design or even solve business problems is changing how business is done. Gen AI’s capacity to generate new data and solutions independently has opened opportunities for advancements as has never been seen before.
If companies are adopting Generative AI , then the next big issue that they are going to meet is on scalability of models and its implementation. These resource- intensive applications present a major challenge to the traditional IT architectures. It is here that Kubernetes comes into the picture, which provides solutions to automate deployment, scaling and managing the containerised applications. Kubernetes may be deployed to facilitate the ML and deep learning processing hence maximizing the efficiency of the AI pipeline to support the future growth of Gen AI applications.
The Intersection of Generative AI and Kubernetes
The integration of Generative AI and Kubernetes is probably the most significant traffic in the development of AI deployment approaches. Kubernetes is perfect for the dynamics of AI workloads in terms of scalability and flexibility. The computation of Gen AI models demands considerable resources, and Kubernetes has all the tools required to properly orchestrate those resources for deploying AI models in different setups.
Kubernetes’ infrastructure is especially beneficial for AI startups and companies that plan to use Generative AI. It enables the decentralization of workload among several nodes so that training, testing, and deployment of AI models are highly distributed. This capability is especially important for businesses that require to constantly revolve their models to adapt to competition. In addition, Kubernetes has direct support for GPU, which helps in evenly distributing computational intensity that comes with deep learning workloads thereby making it perfect for AI projects.
Key Kubernetes Features that Enable Efficient Generative AI Deployment
Scalability:
Kubernetes excels at all levels but most notably where applications are scaled horizontally. Especially for Generative AI which often needs a lot of computation, Kubernetes is capable of scaling the pods, the instances of the running processes and provide necessary resources for the workload claims without having any human intervention.
Resource Management:
Effort is required to be allocated efficiently so as to perform the AI workloads. Kubernetes assists in deploying as well as allocating resources within the cluster from where the AI models usually operate while ensuring that resource consumption and distribution is efficiently controlled.
Continuous Deployment and Integration (CI/CD):
Kubernetes allows for the execution of CI CD pipelines which facilitate contingency integration as well as contingency deployment of models. This is essential for enterprises and the AI startups that use the flexibility of launching different AI solutions depending on the current needs of their companies.
GPU Support:
Kubernetes also features the support of the GPUs for the applications in deep learning from scratch that enhances the rate of training and inference of the models of AI. It is particularly helpful for AI applications that require more data processing, such as image and speech recognition.
Multi-Cloud and Hybrid Cloud Support:
The fact that the Kubernetes can operate in several cloud environment and on-premise data centers makes it versatile as AI deployment tool. It will benefit organizations that need a half and half cloud solution and organizations that do not want to be trapped in the web of the specific company.
Challenges of Running Generative AI on Kubernetes
Complexity of Setup and Management:
That aid Kubernetes provides a great platform for AI deployments comes at the cost of operational overhead. Deploying and configuring a Kubernetes Cluster for AI based workloads therefore necessitates knowledge of both Kubernetes and the approach used to develop these models. This could be an issue for organizations that are not able to gather or hire the required expertise.
Resource Constraints:
Generative AI models require a lot of computing power and when running them in a Kubernetes environment, the computational resources can be fully utilised. AI works best when the organizational resources are well managed to ensure that there are no constraints in the delivery of the application services.
Security Concerns:
Like it is the case with any cloud-native application, security is a big issue when it comes to running artificial intelligence models on Kubernetes. Security of the data and models that AI employs needs to be protected hence comes the policies of encryption, access control and monitoring.
Data Management:
Generative AI models make use of multiple dataset samples for its learning process and is hard to deal with the concept in Kubernetes. Managing these datasets as well as accessing and processing them in a manner that does not hinder the overall performance of an organization is often a difficult task.
Conclusion: The Future of Generative AI is Powered by Kubernetes
As Generative AI advances and integrates into many sectors, the Kubernetes efficient and scalable solutions will only see a higher adoption rate. Kubernetes is a feature of AI architectures that offer resources and facilities for the development and management of AI model deployment.
If you’re an organization planning on putting Generative AI to its best use, then adopting Kubernetes is non-negotiable. Mounting the AI workloads, utilizing the resources in the best possible manner, and maintaining the neat compatibility across the multiple and different clouds are some of the key solutions provided by Kubernetes for the deployment of the AI models. With continued integration between Generative AI and Kubernetes, we have to wonder what new and exciting uses and creations are yet to come, thus strengthening Kubernetes’ position as the backbone for enterprise AI with Kubernetes. The future is bright that Kubernetes is playing leading role in this exciting technological revolution of AI.
Original Source: https://bit.ly/4cPS7G0
#AI Startups Kubernetes#Enterprise AI With Kubernetes#Generative AI#Kubernetes AI Architecture#Kubernetes For AI Model Deployment#Kubernetes For Deep Learning#Kubernetes For Machine Learning
0 notes
Text
How To Use Llama 3.1 405B FP16 LLM On Google Kubernetes
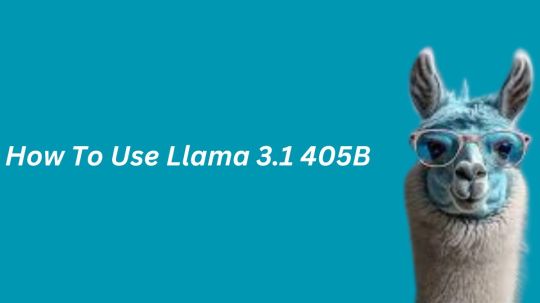
How to set up and use large open models for multi-host generation AI over GKE
Access to open models is more important than ever for developers as generative AI grows rapidly due to developments in LLMs (Large Language Models). Open models are pre-trained foundational LLMs that are accessible to the general population. Data scientists, machine learning engineers, and application developers already have easy access to open models through platforms like Hugging Face, Kaggle, and Google Cloud’s Vertex AI.
How to use Llama 3.1 405B
Google is announcing today the ability to install and run open models like Llama 3.1 405B FP16 LLM over GKE (Google Kubernetes Engine), as some of these models demand robust infrastructure and deployment capabilities. With 405 billion parameters, Llama 3.1, published by Meta, shows notable gains in general knowledge, reasoning skills, and coding ability. To store and compute 405 billion parameters at FP (floating point) 16 precision, the model needs more than 750GB of GPU RAM for inference. The difficulty of deploying and serving such big models is lessened by the GKE method discussed in this article.
Customer Experience
You may locate the Llama 3.1 LLM as a Google Cloud customer by selecting the Llama 3.1 model tile in Vertex AI Model Garden.
Once the deploy button has been clicked, you can choose the Llama 3.1 405B FP16 model and select GKE.Image credit to Google Cloud
The automatically generated Kubernetes yaml and comprehensive deployment and serving instructions for Llama 3.1 405B FP16 are available on this page.
Deployment and servicing multiple hosts
Llama 3.1 405B FP16 LLM has significant deployment and service problems and demands over 750 GB of GPU memory. The total memory needs are influenced by a number of parameters, including the memory used by model weights, longer sequence length support, and KV (Key-Value) cache storage. Eight H100 Nvidia GPUs with 80 GB of HBM (High-Bandwidth Memory) apiece make up the A3 virtual machines, which are currently the most potent GPU option available on the Google Cloud platform. The only practical way to provide LLMs such as the FP16 Llama 3.1 405B model is to install and serve them across several hosts. To deploy over GKE, Google employs LeaderWorkerSet with Ray and vLLM.
LeaderWorkerSet
A deployment API called LeaderWorkerSet (LWS) was created especially to meet the workload demands of multi-host inference. It makes it easier to shard and run the model across numerous devices on numerous nodes. Built as a Kubernetes deployment API, LWS is compatible with both GPUs and TPUs and is independent of accelerators and the cloud. As shown here, LWS uses the upstream StatefulSet API as its core building piece.
A collection of pods is controlled as a single unit under the LWS architecture. Every pod in this group is given a distinct index between 0 and n-1, with the pod with number 0 being identified as the group leader. Every pod that is part of the group is created simultaneously and has the same lifecycle. At the group level, LWS makes rollout and rolling upgrades easier. For rolling updates, scaling, and mapping to a certain topology for placement, each group is treated as a single unit.
Each group’s upgrade procedure is carried out as a single, cohesive entity, guaranteeing that every pod in the group receives an update at the same time. While topology-aware placement is optional, it is acceptable for all pods in the same group to co-locate in the same topology. With optional all-or-nothing restart support, the group is also handled as a single entity when addressing failures. When enabled, if one pod in the group fails or if one container within any of the pods is restarted, all of the pods in the group will be recreated.
In the LWS framework, a group including a single leader and a group of workers is referred to as a replica. Two templates are supported by LWS: one for the workers and one for the leader. By offering a scale endpoint for HPA, LWS makes it possible to dynamically scale the number of replicas.
Deploying multiple hosts using vLLM and LWS
vLLM is a well-known open source model server that uses pipeline and tensor parallelism to provide multi-node multi-GPU inference. Using Megatron-LM’s tensor parallel technique, vLLM facilitates distributed tensor parallelism. With Ray for multi-node inferencing, vLLM controls the distributed runtime for pipeline parallelism.
By dividing the model horizontally across several GPUs, tensor parallelism makes the tensor parallel size equal to the number of GPUs at each node. It is crucial to remember that this method requires quick network connectivity between the GPUs.
However, pipeline parallelism does not require continuous connection between GPUs and divides the model vertically per layer. This usually equates to the quantity of nodes used for multi-host serving.
In order to support the complete Llama 3.1 405B FP16 paradigm, several parallelism techniques must be combined. To meet the model’s 750 GB memory requirement, two A3 nodes with eight H100 GPUs each will have a combined memory capacity of 1280 GB. Along with supporting lengthy context lengths, this setup will supply the buffer memory required for the key-value (KV) cache. The pipeline parallel size is set to two for this LWS deployment, while the tensor parallel size is set to eight.
In brief
We discussed in this blog how LWS provides you with the necessary features for multi-host serving. This method maximizes price-to-performance ratios and can also be used with smaller models, such as the Llama 3.1 405B FP8, on more affordable devices. Check out its Github to learn more and make direct contributions to LWS, which is open-sourced and has a vibrant community.
You can visit Vertex AI Model Garden to deploy and serve open models via managed Vertex AI backends or GKE DIY (Do It Yourself) clusters, as the Google Cloud Platform assists clients in embracing a gen AI workload. Multi-host deployment and serving is one example of how it aims to provide a flawless customer experience.
Read more on Govindhtech.com
#Llama3.1#Llama#LLM#GoogleKubernetes#GKE#405BFP16LLM#AI#GPU#vLLM#LWS#News#Technews#Technology#Technologynews#Technologytrends#govindhtech
2 notes
·
View notes
Text
How to Choose the Right Netflix Clone Development Partner
The demand for OTT (Over-the-Top) platforms has surged dramatically, with streaming services becoming a staple of global entertainment. As entrepreneurs and media companies seek to tap into this digital revolution, many opt to launch platforms modeled on successful giants like Netflix. A popular approach is building a Netflix clone—a customized video streaming platform with core functionalities similar to Netflix. However, the success of such a venture depends heavily on selecting the right development partner. This essay provides a step-by-step guide to choosing the best Netflix clone development company to bring your streaming vision to life.
1. Define Your Business Objectives
Before beginning your search, clearly define what you want from your Netflix clone:
Do you need a fully custom OTT solution or a white-label product?
Will your platform target a global audience or a niche regional market?
What monetization model will you use—subscriptions, ads, or pay-per-view?
Understanding your vision will help you choose a partner whose expertise aligns with your business goals.
2. Evaluate Technical Expertise
Your development partner should have a proven track record in OTT app development. Check if the company:
Uses modern, scalable technologies (e.g., React, Node.js, AWS, Kubernetes).
Offers video streaming features like adaptive bitrate, CDN integration, and DRM.
Provides a robust admin dashboard and user analytics tools.
Supports multi-platform delivery (Android, iOS, web, smart TV).
Technical depth ensures your Netflix clone performs seamlessly under real-world conditions.
3. Assess Experience in the OTT Space
Not all app developers specialize in streaming platforms. Look for:
Portfolio of past OTT projects or Netflix clones.
Client reviews and testimonials specifically related to video streaming.
Case studies that show real-world problem-solving in the media domain.
OTT experience means they’ll understand challenges like content delivery, copyright management, and user engagement strategies.
4. Prioritize Customization Capabilities
Every business is unique. Avoid cookie-cutter solutions that limit growth or differentiation. Choose a company that:
Offers full customization of UI/UX, content categories, and features.
Supports third-party integrations (e.g., payment gateways, analytics, marketing tools).
Can scale features as your user base grows (e.g., live streaming, AI-based recommendations).
Customizability is essential for long-term platform success and brand identity.
5. Ensure End-to-End Services
A reliable partner should provide end-to-end OTT development services, including:
UI/UX design
Backend and frontend development
Hosting and deployment
Post-launch support and maintenance
App updates and feature enhancements
Comprehensive service saves you from dealing with multiple vendors and reduces coordination issues.
6. Consider Cost vs. Value
Budget is important, but don’t make it your only criteria. Low-cost solutions often come with compromises in quality or scalability. Evaluate:
What’s included in their pricing (e.g., mobile apps, CMS, monetization tools)?
Are there hidden fees for updates, third-party services, or tech support?
Is there a flexible payment plan or milestone-based pricing?
Choose a partner that offers the best value for your investment, not just the cheapest option.
7. Check Support & Maintenance Options
Post-launch support is critical for any OTT platform. Confirm that your development partner provides:
24/7 customer support and bug resolution
Timely app updates based on new devices or OS changes
Continuous performance optimization
Long-term maintenance ensures your platform remains secure, fast, and relevant.
8. Ask About Security & Compliance
Streaming platforms handle sensitive user data and copyrighted content. Your development partner should:
Follow industry best practices for data security and encryption.
Comply with international privacy laws (e.g., GDPR, COPPA).
Offer DRM (Digital Rights Management) integration to protect content.
Security builds user trust and protects your business from legal issues.
9. Request a Demo or Free Trial
Before committing, ask for a live demo of their Netflix clone or a free trial. This allows you to:
Test the performance and UI firsthand.
Evaluate the content management system (CMS).
Understand the customization scope and technical limitations.
A hands-on experience is often more revealing than sales presentations.
10. Review Communication and Project Management
Good communication ensures smooth collaboration. Ensure the company:
Assigns a dedicated project manager.
Uses project management tools like Jira, Trello, or Asana.
Offers clear timelines, progress reports, and milestone tracking.
Strong project management results in timely delivery and fewer surprises.
Conclusion
Choosing the right Netflix clone development partner is a pivotal decision that can define your OTT platform's success. By evaluating technical capabilities, industry experience, customization options, support services, and communication standards, you can align with a development team that not only understands your vision but also has the tools and experience to make it a reality. In the fast-paced world of digital entertainment, choosing wisely today ensures you remain competitive tomorrow.
0 notes
Text
Generative AI in IT Workspace: The Future of Real-Time Decision-Making, Downtime Prevention, and Infrastructure Optimization
The role of IT in enterprise operations has evolved beyond managing infrastructure. Today, IT is at the heart of every strategic initiative—from enabling remote work and maintaining cybersecurity to ensuring seamless customer experiences. Amidst this complexity, Generative AI in the IT workspace is emerging as a transformative force. It empowers teams to shift from reactive problem-solving to proactive and predictive operations.
This blog explores how Generative AI is revolutionizing IT environments through real-time decision-making, minimizing downtime, and optimizing infrastructure for scalable, intelligent operations.
1. Understanding Generative AI in the Context of IT Operations
Generative AI is not just about generating text or code—it’s about simulating reasoning, pattern recognition, and intelligent action. In the IT workspace, this translates to:
Generating actionable insights from vast, unstructured logs
Creating automated scripts for issue resolution
Simulating infrastructure changes before deployment
Learning from past incidents to prevent future failures
Unlike traditional AI models focused on classification or regression, Generative AI builds upon large language models (LLMs) and foundation models to perform autonomous tasks, suggest solutions, and dynamically evolve with the IT ecosystem.
2. Real-Time Decision-Making Powered by Generative AI
Why Real-Time Matters
Milliseconds matter in IT—whether it’s detecting a DDoS attack or reallocating cloud resources to meet demand spikes. Real-time decision-making improves:
Incident response speed
System reliability
End-user satisfaction
How Generative AI Makes It Happen
Conversational Interfaces: AI copilots integrated into tools like Microsoft Teams or Slack provide real-time alerts, recommendations, and command execution capabilities.
Streaming Data Analysis: Generative AI processes live telemetry and logs, summarizing anomalies or performance trends instantly.
Context-Aware Actions: The AI understands current configurations, dependencies, and SLAs to offer decisions aligned with business impact.
Example: If a database shows high latency, the AI might suggest a query optimization, initiate a failover, or provision additional compute resources—automatically or with human approval.
3. Downtime Prevention Through Predictive and Autonomous Action
The High Cost of Downtime
Downtime costs businesses an average of $5,600 per minute, according to Gartner. From lost revenue to reputational damage, the consequences are severe. Prevention requires foresight—and this is where Generative AI excels.
Key Capabilities of Generative AI in Downtime Prevention
Anomaly Detection with Root Cause Suggestions AI agents can analyze historical outages and detect early warning signs, offering probable causes and quick fixes before issues escalate.
Predictive Maintenance of Infrastructure By learning patterns in system degradation, AI can forecast component failures—whether it’s a memory leak, disk I/O bottleneck, or CPU overheating.
Self-Healing Systems Generative AI can autonomously trigger scripts to restart services, rebalance workloads, or isolate affected containers without waiting for human input.
Real-world use case: In Kubernetes environments, AI agents can monitor node health and proactively reschedule workloads from a failing pod to prevent service disruption.
4. Infrastructure Optimization for Efficiency and Scalability
Why Optimization Is a Moving Target
In cloud-native environments, optimization isn’t a one-time task—it’s a continuous loop. You’re balancing cost, performance, latency, and availability across hybrid or multi-cloud setups. Generative AI simplifies this complexity.
What Generative AI Does Differently
Dynamic Resource Allocation: Based on real-time application load and user behavior, AI recommends or initiates scaling operations—horizontal or vertical.
Configuration Tuning: From serverless functions to container orchestration, the AI tunes parameters like memory limits, concurrency thresholds, and storage policies.
Cloud Cost Intelligence: Generative AI generates contextual cost breakdowns and optimization strategies (e.g., moving workloads to spot instances or lower-tier storage).
Impact: IT leaders can expect 20–30% cost reduction and 40% better resource utilization with AI-powered infrastructure insights.
5. Generative AI Agents as Digital Co-Pilots for IT Teams
Generative AI isn't replacing IT professionals—it’s augmenting their capabilities. AI copilots embedded in the workspace offer:
Task Automation: From provisioning virtual machines to rolling out security patches.
Knowledge Retrieval: Pulling relevant SOPs, architecture diagrams, or config files in seconds.
Incident Collaboration: Summarizing events, compiling response timelines, and suggesting RCA documentation.
The result? Faster resolution, reduced human error, and a smarter IT workforce.
6. Seamless Integration Across ITSM, DevOps, and Monitoring Tools
Generative AI platforms can be integrated with:
ITSM platforms like ServiceNow and Zendesk
Monitoring tools like Datadog, Prometheus, and New Relic
CI/CD pipelines in GitLab, Jenkins, or Azure DevOps
Once connected, the AI doesn't just observe—it acts:
Auto-generates tickets from alerts
Suggests remediation based on past fixes
Adds intelligent context to every incident report
This fusion enables closed-loop automation—from issue detection to ticket resolution, entirely streamlined.
7. Security, Governance, and Human Oversight
Adopting Generative AI in IT demands a strong foundation of:
Data privacy and access controls
AI model explainability and auditing
Fail-safes and human-in-the-loop mechanisms
With the right governance, organizations can ensure that AI recommendations align with compliance frameworks like ISO 27001, NIST, or GDPR.
8. Business Impact and ROI of Generative AI in IT
According to a McKinsey report (2024), companies that embedded Generative AI into their IT operations saw:
60% reduction in mean time to resolution (MTTR)
35% improvement in system uptime
3x increase in employee productivity for Level 1 support
When measured over a year, the ROI is substantial—both in dollars and in strategic agility.
Conclusion: The AI-Driven IT Future Is Here
As businesses demand always-on services, secure platforms, and lightning-fast innovation, IT teams can no longer rely on manual operations alone. Generative AI in the IT workspace is the intelligent engine that powers real-time decisions, prevents downtime, and optimizes infrastructure—continuously.
It’s not a tool of the future—it’s the competitive advantage of today.
0 notes
Text
A-SWE AI Agent: OpenAI's Vision for Replacing Human Developers

OpenAI has made yet another groundbreaking announcement—introducing the A-SWE AI Agent, a fully autonomous AI system designed to revolutionize software development. This cutting-edge agent promises to replace traditional human software engineers by automating the entire software development lifecycle, including tasks such as coding, testing, deployment, and debugging. The A-SWE AI Agent represents a bold leap toward a future where AI is not just a tool but a creator in its own right. As a CAIE Certified Artificial Intelligence (AI) Expert®, Certified Cyber Security Expert™, Certified Network Security Engineer™, Certified Python Developer™, and holder of a Machine Learning Certification, I will explore the technological significance, potential applications, and broader implications of this development.
What Is the A-SWE AI Agent?
The A-SWE AI Agent is an advanced artificial intelligence system developed by OpenAI to autonomously perform software engineering tasks. Unlike traditional AI assistants that require human input for every action, the A-SWE agent operates independently, analyzing user requirements, writing code, debugging errors, and even testing applications.
A-SWE stands for Agentic Software Engineer, highlighting its focus on fully autonomous software development. Using state-of-the-art machine learning algorithms, natural language processing, and advanced code generation models, the A-SWE agent can manage complex software projects without constant human intervention. It takes on the role of the software engineer, handling the routine and often time-consuming tasks that typically require human input.
Key Features of the A-SWE AI Agent
Autonomous Code Generation The A-SWE AI Agent is capable of writing clean, efficient, and optimized code based on user specifications. By leveraging advanced language models and pre-trained data, it can analyze project requirements, understand the programming language needed, and generate functional code autonomously. This capability allows developers to save significant time in the coding phase.
Debugging and Testing Debugging is often one of the most tedious parts of software development. The A-SWE agent can autonomously detect errors in code, suggest fixes, and even implement them. In addition, it can generate and run test cases, ensuring that the software is robust, secure, and bug-free before deployment. This feature reduces human error and speeds up the overall testing process.
Seamless Integration with Development Tools A-SWE is designed to work seamlessly with popular development tools and platforms such as GitHub, Docker, and Kubernetes. By integrating with these systems, it can manage version control, automate deployments, and facilitate continuous integration/continuous deployment (CI/CD) pipelines. This makes it easier for developers to integrate the AI agent into existing workflows.
Optimized Code Refactoring Code refactoring—improving the internal structure of code without changing its external behavior—is a critical task in software development. The A-SWE agent can autonomously refactor code to improve efficiency, reduce redundancy, and ensure scalability. This feature enables the agent to maintain high-quality, performant code as projects evolve.
Smart Decision-Making A-SWE can make informed decisions about the best course of action based on predefined goals or project specifications. This decision-making ability enables the agent to prioritize tasks, allocate resources, and even provide suggestions on how to solve complex programming challenges.
Potential Applications of the A-SWE AI Agent
The introduction of the A-SWE AI Agent has vast implications for various industries. Here are a few key areas where this AI technology could have a profound impact:
Software Development The most obvious application of A-SWE is in software development itself. By automating routine tasks like coding, debugging, and testing, the AI agent can free human developers to focus on more strategic aspects of development. It also significantly reduces the time required to build and deploy software applications, leading to faster turnaround times and more efficient workflows.
Enterprise IT Automation Many businesses rely on custom-built software applications to meet their specific needs. The A-SWE AI Agent can automate the development and maintenance of these applications, reducing the need for in-house developers and allowing organizations to focus on innovation. It can also assist in maintaining and updating legacy systems.
Open-Source Development The A-SWE AI Agent could boost open-source software development. The agent can autonomously contribute to open-source projects by writing code, fixing bugs, and optimizing features, helping to accelerate the development of community-driven software.
Education and Training The A-SWE agent has the potential to transform education in software engineering. By serving as an AI-powered tutor, it can teach students how to write clean, efficient code and provide real-time feedback on their work. It can also help train new developers by offering code examples and explanations, making it easier for beginners to learn programming.
Implications for the Tech Industry
The launch of the A-SWE AI Agent represents a monumental shift in the way software is developed. As AI continues to take over more complex tasks, the role of human software engineers will inevitably evolve. Developers will likely focus on higher-level design, architecture, and decision-making while leaving routine tasks to the AI agent.
This shift could have profound implications for the tech industry:
Job Displacement: While AI can improve efficiency, it also raises concerns about job displacement. Many junior developers, whose primary tasks revolve around writing code and debugging, may face reduced job opportunities as A-SWE becomes more capable.
Skills Development: On the other hand, the rise of AI in software development will create new opportunities for upskilling. Developers will need to learn how to collaborate with AI systems, manage AI-driven development processes, and handle more complex problem-solving tasks. Certifications like Certified Python Developer™ Certification and Machine Learning Certification will become increasingly important for those looking to stay ahead of the curve.
Ethical Considerations: The widespread adoption of autonomous AI agents in software development also raises ethical concerns, particularly around algorithmic bias, data privacy, and accountability. Ensuring that AI systems like A-SWE are transparent, fair, and secure will be critical as they become more integrated into the development process.
Preparing for the AI-Driven Future
As AI continues to evolve, tech industry professionals will need to adapt to this new landscape. Pursuing certifications such as Certified Python Developer™ Certification, Machine Learning Certification, and Certified Cyber Security Expert™ Certification will be essential for those looking to understand and utilize AI technologies effectively.
Additionally, professionals with expertise in network security, such as those with a Certified Network Security Engineer™ Certification, will be needed to ensure that AI systems like A-SWE are secure and protected from malicious attacks. As AI agents handle more critical tasks, ensuring their integrity will become increasingly important.
Conclusion
OpenAI’s A-SWE AI Agent is poised to transform the software development industry by automating tasks traditionally performed by human developers. With its ability to generate code, debug software, and handle testing autonomously, A-SWE promises to revolutionize how applications are created and maintained. While this advancement presents numerous opportunities for efficiency and innovation, it also brings challenges related to job displacement, ethical concerns, and security.For professionals looking to stay competitive in this AI-driven future, acquiring relevant certifications, such as Certified Python Developer™ Certification, Machine Learning Certification, and Certified Cyber Security Expert™ Certification, will be essential for developing the necessary skills to navigate this new technological landscape. The rise of AI in software development is not just about automation but also about collaboration between humans and AI, creating a more efficient, innovative, and secure future for the tech industry.
0 notes
Text
Google Cloud Solution: Empowering Businesses with Scalable Innovation
In today’s fast-paced digital era, cloud computing has become the cornerstone of innovation and operational efficiency. At Izoe Solution, we harness the power of Google Cloud Platform (GCP) to deliver robust, scalable, and secure cloud solutions that drive business transformation across industries.
Why Google Cloud?
Google Cloud offers a comprehensive suite of cloud services that support a wide range of enterprise needs—from data storage and computing to advanced AI/ML capabilities. Known for its powerful infrastructure, cutting-edge tools, and strong security framework, Google Cloud is trusted by some of the world's largest organizations.
Our Core Google Cloud Services
Cloud Migration & Modernization We help businesses migrate their workloads and applications to Google Cloud with minimal disruption. Our phased approach ensures data integrity, performance tuning, and post-migration optimization.
Data Analytics & BigQuery Izoe Solution leverages Google’s powerful BigQuery platform to deliver real-time analytics, enabling data-driven decision-making. We build end-to-end data pipelines for maximum business insight.
App Development & Hosting Using Google App Engine, Kubernetes Engine, and Cloud Functions, we develop and deploy modern applications that are secure, scalable, and cost-efficient.
AI and Machine Learning From image recognition to predictive modeling, we implement Google Cloud AI/ML tools like Vertex AI to build intelligent systems that enhance customer experiences and operational efficiency.
Cloud Security & Compliance Security is at the core of every cloud solution we design. We follow best practices and leverage Google’s built-in security features to ensure data protection, access control, and compliance with industry standards.
Why Choose Izoe Solution?
Certified Google Cloud Professionals: Our team holds GCP certifications and brings hands-on expertise in architecture, development, and operations.
Tailored Solutions: We design cloud architectures that align with your unique business goals.
End-to-End Support: From planning and deployment to monitoring and optimization, we provide continuous support throughout your cloud journey.
Proven Results: Our solutions have improved performance, reduced costs, and accelerated innovation for clients across sectors.
Conclusion
Cloud adoption is no longer optional—it's essential. Partner with Izoe Solution to leverage the full potential of Google Cloud and future-proof your business with intelligent, secure, and scalable solutions.
Contact us today to learn how Izoe Solution can transform your business through Google Cloud.
0 notes
Text
Hybrid Cloud Application: The Smart Future of Business IT
Introduction
In today’s digital-first environment, businesses are constantly seeking scalable, flexible, and cost-effective solutions to stay competitive. One solution that is gaining rapid traction is the hybrid cloud application model. Combining the best of public and private cloud environments, hybrid cloud applications enable businesses to maximize performance while maintaining control and security.
This 2000-word comprehensive article on hybrid cloud applications explains what they are, why they matter, how they work, their benefits, and how businesses can use them effectively. We also include real-user reviews, expert insights, and FAQs to help guide your cloud journey.
What is a Hybrid Cloud Application?
A hybrid cloud application is a software solution that operates across both public and private cloud environments. It enables data, services, and workflows to move seamlessly between the two, offering flexibility and optimization in terms of cost, performance, and security.
For example, a business might host sensitive customer data in a private cloud while running less critical workloads on a public cloud like AWS, Azure, or Google Cloud Platform.
Key Components of Hybrid Cloud Applications
Public Cloud Services – Scalable and cost-effective compute and storage offered by providers like AWS, Azure, and GCP.
Private Cloud Infrastructure – More secure environments, either on-premises or managed by a third-party.
Middleware/Integration Tools – Platforms that ensure communication and data sharing between cloud environments.
Application Orchestration – Manages application deployment and performance across both clouds.
Why Choose a Hybrid Cloud Application Model?
1. Flexibility
Run workloads where they make the most sense, optimizing both performance and cost.
2. Security and Compliance
Sensitive data can remain in a private cloud to meet regulatory requirements.
3. Scalability
Burst into public cloud resources when private cloud capacity is reached.
4. Business Continuity
Maintain uptime and minimize downtime with distributed architecture.
5. Cost Efficiency
Avoid overprovisioning private infrastructure while still meeting demand spikes.
Real-World Use Cases of Hybrid Cloud Applications
1. Healthcare
Protect sensitive patient data in a private cloud while using public cloud resources for analytics and AI.
2. Finance
Securely handle customer transactions and compliance data, while leveraging the cloud for large-scale computations.
3. Retail and E-Commerce
Manage customer interactions and seasonal traffic spikes efficiently.
4. Manufacturing
Enable remote monitoring and IoT integrations across factory units using hybrid cloud applications.
5. Education
Store student records securely while using cloud platforms for learning management systems.
Benefits of Hybrid Cloud Applications
Enhanced Agility
Better Resource Utilization
Reduced Latency
Compliance Made Easier
Risk Mitigation
Simplified Workload Management
Tools and Platforms Supporting Hybrid Cloud
Microsoft Azure Arc – Extends Azure services and management to any infrastructure.
AWS Outposts – Run AWS infrastructure and services on-premises.
Google Anthos – Manage applications across multiple clouds.
VMware Cloud Foundation – Hybrid solution for virtual machines and containers.
Red Hat OpenShift – Kubernetes-based platform for hybrid deployment.
Best Practices for Developing Hybrid Cloud Applications
Design for Portability Use containers and microservices to enable seamless movement between clouds.
Ensure Security Implement zero-trust architectures, encryption, and access control.
Automate and Monitor Use DevOps and continuous monitoring tools to maintain performance and compliance.
Choose the Right Partner Work with experienced providers who understand hybrid cloud deployment strategies.
Regular Testing and Backup Test failover scenarios and ensure robust backup solutions are in place.
Reviews from Industry Professionals
Amrita Singh, Cloud Engineer at FinCloud Solutions:
"Implementing hybrid cloud applications helped us reduce latency by 40% and improve client satisfaction."
John Meadows, CTO at EdTechNext:
"Our LMS platform runs on a hybrid model. We’ve achieved excellent uptime and student experience during peak loads."
Rahul Varma, Data Security Specialist:
"For compliance-heavy environments like finance and healthcare, hybrid cloud is a no-brainer."
Challenges and How to Overcome Them
1. Complex Architecture
Solution: Simplify with orchestration tools and automation.
2. Integration Difficulties
Solution: Use APIs and middleware platforms for seamless data exchange.
3. Cost Overruns
Solution: Use cloud cost optimization tools like Azure Advisor, AWS Cost Explorer.
4. Security Risks
Solution: Implement multi-layered security protocols and conduct regular audits.
FAQ: Hybrid Cloud Application
Q1: What is the main advantage of a hybrid cloud application?
A: It combines the strengths of public and private clouds for flexibility, scalability, and security.
Q2: Is hybrid cloud suitable for small businesses?
A: Yes, especially those with fluctuating workloads or compliance needs.
Q3: How secure is a hybrid cloud application?
A: When properly configured, hybrid cloud applications can be as secure as traditional setups.
Q4: Can hybrid cloud reduce IT costs?
A: Yes. By only paying for public cloud usage as needed, and avoiding overprovisioning private servers.
Q5: How do you monitor a hybrid cloud application?
A: With cloud management platforms and monitoring tools like Datadog, Splunk, or Prometheus.
Q6: What are the best platforms for hybrid deployment?
A: Azure Arc, Google Anthos, AWS Outposts, and Red Hat OpenShift are top choices.
Conclusion: Hybrid Cloud is the New Normal
The hybrid cloud application model is more than a trend—it’s a strategic evolution that empowers organizations to balance innovation with control. It offers the agility of the cloud without sacrificing the oversight and security of on-premises systems.
If your organization is looking to modernize its IT infrastructure while staying compliant, resilient, and efficient, then hybrid cloud application development is the way forward.
At diglip7.com, we help businesses build scalable, secure, and agile hybrid cloud solutions tailored to their unique needs. Ready to unlock the future? Contact us today to get started.
0 notes
Text
Unveil how Gen AI is pushing Kubernetes to the forefront, delivering industry-specific solutions with precision and scalability.
#AI Startups Kubernetes#Enterprise AI With Kubernetes#Generative AI#Kubernetes AI Architecture#Kubernetes For AI Model Deployment#Kubernetes For Deep Learning#Kubernetes For Machine Learning
0 notes
Text
Best Software Development Company in Chennai: Your Partner for Digital Excellence

In today’s fast-paced digital landscape, partnering with the best software development company in Chennai can be the key to transforming your business vision into reality. Chennai, a thriving IT hub, is home to numerous firms specializing in cutting-edge technologies—from AI and blockchain to cloud-native applications. Whether you’re a startup seeking an MVP or an enterprise ready for digital transformation, choosing the right Software Development Company in Chennai ensures top-tier quality, on‑time delivery, and scalable solutions.
Why Choose a Software Development Company in Chennai?
Rich IT Ecosystem Chennai boasts a vibrant ecosystem of skilled engineers, designers, and project managers. The city’s robust educational institutions and thriving tech parks cultivate talent proficient in the latest programming languages and development frameworks.
Cost-Effective Excellence Compared to Western markets, Chennai offers highly competitive rates without compromising on code quality or innovation. This cost advantage enables businesses of all sizes to access world‑class software solutions within budget.
Agile & Customer‑Centric Approach Leading firms in Chennai adopt Agile methodologies—breaking projects into sprints, facilitating continuous feedback loops, and ensuring that deliverables align precisely with client expectations.
Strong Communication & Support With English as the primary medium and overlapping work hours with Europe and parts of Asia, Chennai teams maintain clear, real‑time communication, seamless collaboration, and dependable post‑launch support.
Core Services Offered
A top Software Development Company in Chennai typically provides:
Custom Software Development: Tailor‑made applications powered by Java, .NET, Python, or Node.js to meet your unique business requirements.
Mobile App Development: Native and cross‑platform apps built with Swift, Kotlin, React Native, or Flutter for iOS and Android.
Web Application Development: Responsive, secure, and SEO‑friendly web portals using Angular, React, Vue.js, or Laravel.
Enterprise Solutions: Scalable ERP, CRM, and BI tools that optimize operations and provide actionable insights.
Cloud Services & DevOps: AWS, Azure, or Google Cloud deployments paired with CI/CD pipelines—ensuring high availability, security, and rapid releases.
UI/UX Design: Intuitive interfaces and immersive user experiences guided by data‑driven design principles and user testing.
Technology Stack & Expertise
Front‑End: React, Angular, Vue.js, Svelte
Back‑End: Node.js, Django, Spring Boot, .NET Core
Databases: MySQL, PostgreSQL, MongoDB, Redis
Mobile: Flutter, React Native, Swift, Kotlin
Cloud & DevOps: Docker, Kubernetes, Jenkins, Terraform, AWS, Azure, GCP
Emerging Tech: AI/ML models in TensorFlow and PyTorch, Blockchain development, IoT integrations
Our Proven Process
Discovery & Planning
Stakeholder workshops to define scope
Requirement analysis and feasibility studies
Project roadmap with milestones and timelines
Design & Prototyping
Wireframes and interactive mockups
UI/UX validation through user feedback
Design handoff with detailed style guides
Development & Iteration
Agile sprints with regular demos
Continuous integration and code reviews
Unit, integration, and performance testing
Quality Assurance
Automated and manual testing for functionality and security
Compatibility checks across devices and browsers
Load testing to ensure scalability
Deployment & Maintenance
Staged releases: dev → staging → production
24/7 monitoring, troubleshooting, and updates
Dedicated support plans for ongoing enhancements
Success Stories
FinTech Startup: Developed a real‑time trading platform with React and Node.js, supporting over 10,000 concurrent users and reducing transaction latency by 40%. Healthcare Portal: Created a HIPAA‑compliant patient management system on Azure, improving appointment scheduling efficiency by 60%. E‑Learning Platform: Built a scalable LMS with Laravel and Vue.js, accommodating 50,000+ users and integrating interactive video lectures.
Why We Stand Out
Client‑First Culture: Transparent reporting, flexible engagement models (T&M, fixed‑price, dedicated teams), and a commitment to your success.
Certified Experts: AWS Solution Architects, Microsoft Gold Partners, and Scrum Masters drive every project.
Innovation Labs: R&D teams exploring AI, blockchain, and VR to keep you ahead of the curve.
Quality Assurance: ISO 9001 and CMMI Level 3 certifications ensure rigorous process adherence.
Conclusion & Next Steps
Selecting the best software development company in Chennai means partnering with a team that blends technical prowess, creative design, and unwavering dedication to your goals. Ready to accelerate your digital journey? Get in touch today for a free consultation and project estimate with our award‑winning Software Development Company in Chennai. Let’s build the future—together.
0 notes
Text
Creating and Configuring Production ROSA Clusters (CS220) – A Practical Guide
Introduction
Red Hat OpenShift Service on AWS (ROSA) is a powerful managed Kubernetes solution that blends the scalability of AWS with the developer-centric features of OpenShift. Whether you're modernizing applications or building cloud-native architectures, ROSA provides a production-grade container platform with integrated support from Red Hat and AWS. In this blog post, we’ll walk through the essential steps covered in CS220: Creating and Configuring Production ROSA Clusters, an instructor-led course designed for DevOps professionals and cloud architects.
What is CS220?
CS220 is a hands-on, lab-driven course developed by Red Hat that teaches IT teams how to deploy, configure, and manage ROSA clusters in a production environment. It is tailored for organizations that are serious about leveraging OpenShift at scale with the operational convenience of a fully managed service.
Why ROSA for Production?
Deploying OpenShift through ROSA offers multiple benefits:
Streamlined Deployment: Fully managed clusters provisioned in minutes.
Integrated Security: AWS IAM, STS, and OpenShift RBAC policies combined.
Scalability: Elastic and cost-efficient scaling with built-in monitoring and logging.
Support: Joint support model between AWS and Red Hat.
Key Concepts Covered in CS220
Here’s a breakdown of the main learning outcomes from the CS220 course:
1. Provisioning ROSA Clusters
Participants learn how to:
Set up required AWS permissions and networking pre-requisites.
Deploy clusters using Red Hat OpenShift Cluster Manager (OCM) or CLI tools like rosa and oc.
Use STS (Short-Term Credentials) for secure cluster access.
2. Configuring Identity Providers
Learn how to integrate Identity Providers (IdPs) such as:
GitHub, Google, LDAP, or corporate IdPs using OpenID Connect.
Configure secure, role-based access control (RBAC) for teams.
3. Networking and Security Best Practices
Implement private clusters with public or private load balancers.
Enable end-to-end encryption for APIs and services.
Use Security Context Constraints (SCCs) and network policies for workload isolation.
4. Storage and Data Management
Configure dynamic storage provisioning with AWS EBS, EFS, or external CSI drivers.
Learn persistent volume (PV) and persistent volume claim (PVC) lifecycle management.
5. Cluster Monitoring and Logging
Integrate OpenShift Monitoring Stack for health and performance insights.
Forward logs to Amazon CloudWatch, ElasticSearch, or third-party SIEM tools.
6. Cluster Scaling and Updates
Set up autoscaling for compute nodes.
Perform controlled updates and understand ROSA’s maintenance policies.
Use Cases for ROSA in Production
Modernizing Monoliths to Microservices
CI/CD Platform for Agile Development
Data Science and ML Workflows with OpenShift AI
Edge Computing with OpenShift on AWS Outposts
Getting Started with CS220
The CS220 course is ideal for:
DevOps Engineers
Cloud Architects
Platform Engineers
Prerequisites: Basic knowledge of OpenShift administration (recommended: DO280 or equivalent experience) and a working AWS account.
Course Format: Instructor-led (virtual or on-site), hands-on labs, and guided projects.
Final Thoughts
As more enterprises adopt hybrid and multi-cloud strategies, ROSA emerges as a strategic choice for running OpenShift on AWS with minimal operational overhead. CS220 equips your team with the right skills to confidently deploy, configure, and manage production-grade ROSA clusters — unlocking agility, security, and innovation in your cloud-native journey.
Want to Learn More or Book the CS220 Course? At HawkStack Technologies, we offer certified Red Hat training, including CS220, tailored for teams and enterprises. Contact us today to schedule a session or explore our Red Hat Learning Subscription packages. www.hawkstack.com
0 notes
Text
The Future of Full-Stack Web Development: Trends, Tools, and Technologies to Watch
In the ever-evolving world of tech, few areas have seen as much rapid growth and transformation as full stack web development. What used to be a clear separation between frontend and backend has now turned into a more seamless, hybrid model, where developers are expected to juggle both ends of the spectrum. But where is this all heading?
As we look into the future of full-stack web development, it's clear that exciting changes are on the horizon — from smarter tools and frameworks to revolutionary technologies that promise to redefine how we build for the web. If you're a developer, student, or tech enthusiast, it's time to pay attention.
What is Full Stack Web Development?
Before diving into future trends, let’s briefly revisit what full stack web development really means. A full stack developer works on both:
Frontend (client-side): Everything users interact with — HTML, CSS, JavaScript, and UI frameworks like React or Vue.js.
Backend (server-side): Databases, servers, APIs, and the business logic behind the scenes using technologies like Node.js, Python, Ruby, or Java.
A full stack developer is essentially a digital Swiss Army knife — versatile, adaptable, and always in demand.
Emerging Trends in Full Stack Web Development
Here’s what’s shaping the future:
1. The Rise of Jamstack
Jamstack (JavaScript, APIs, and Markup) is becoming the preferred architecture for faster, more secure, and scalable web applications. Unlike traditional stacks, Jamstack decouples the frontend from the backend, improving performance and simplifying development.
2. AI-Powered Development Tools
Artificial Intelligence is now making its way into code editors and development platforms. Think GitHub Copilot or ChatGPT. These tools assist in writing code, identifying bugs, and even generating entire functions — speeding up the full stack workflow.
Benefits:
Faster coding with AI suggestions
Error prediction and debugging assistance
Smart documentation generation
3. Serverless and Edge Computing
Forget managing traditional servers — serverless architectures and edge computing are becoming the new standard. They allow developers to deploy applications with minimal infrastructure concerns, focusing purely on code and performance.
4. Component-Based Development
Modern frontend frameworks like React, Angular, and Vue are pushing developers towards building reusable components. This modular approach is now extending into the backend too, creating consistent development patterns across the stack.
Tools to Watch in Full Stack Development
To stay relevant, developers must keep their toolkits updated. Here are some must-watch tools shaping the future:
Frontend Tools
React (with Next.js) – For server-side rendering and static generation
Svelte – Lightweight and highly efficient for reactive apps
Tailwind CSS – Utility-first CSS framework for rapid UI development
Backend Tools
Node.js – Continues to dominate with asynchronous performance
Deno – A secure runtime from Node.js’s creator
GraphQL – Replacing REST APIs with a more flexible query language
DevOps & Hosting
Vercel & Netlify – Leading platforms for seamless frontend deployment
Docker & Kubernetes – For containerization and orchestration
Firebase & Supabase – Backend-as-a-service options for fast prototyping
Key Technologies Shaping the Future
Let’s look at the bigger innovations redefining full stack web development:
WebAssembly (WASM): Bringing languages like C++ and Rust to the web browser
Progressive Web Apps (PWAs): Combining web and native app experiences
Blockchain Integration: Decentralized apps (dApps) and smart contract backends
Real-Time Web with WebSockets & MQTT: Enabling live updates and chats
The Human Side of Full Stack Development
Beyond the code and tools, the role of a full stack developer is evolving on a human level too.
Collaborative Skills: Developers must now work more closely with designers, DevOps, and data teams.
Soft Skills Matter: Communication, problem-solving, and adaptability are becoming just as crucial as technical expertise.
Lifelong Learning: With new frameworks emerging almost monthly, continuous learning is a non-negotiable part of the job.
Final Thoughts
Full stack web development is no longer just about knowing a few languages. It’s about understanding entire ecosystems, embracing new paradigms, and building applications that are fast, scalable, and user-centric.
As we look to the future, the lines between frontend and backend will continue to blur, AI will become a coding partner, and developers will be more empowered than ever before. Staying curious, adaptable, and open to learning will be the key to thriving in this dynamic field.
In summary, here’s what to watch for in full stack web development:
Greater automation through AI tools
Continued growth of Jamstack and serverless models
Wider adoption of real-time, decentralized, and modular systems
The rise of multi-disciplinary developer roles
The future is full of possibilities — and if you’re a developer today, you’re right at the center of this exciting evolution.
0 notes
Text
Step-by-Step Guide to Hiring an MLOps Engineer
: Steps to Hire an MLOps Engineer Make the role clear.
Decide your needs: model deployment, CI/CD for ML, monitoring, cloud infrastructure, etc.
2. Choose the level (junior, mid, senior) depending on how advanced the project is.
Create a concise job description.
Include responsibilities like:
2. ML workflow automation (CI/CD)
3. Model lifecycle management (training to deployment)
4. Model performance tracking
5. Utilizing Docker, Kubernetes, Airflow, MLflow, etc.
: Emphasize necessary experience with ML libraries (TensorFlow, PyTorch), cloud platforms (AWS, GCP, Azure), and DevOps tools.
: Source Candidates
Utilize dedicated platforms: LinkedIn, Stack Overflow, GitHub, and AI/ML forums (e.g., MLOps Community, Weights & Biases forums).
Use freelancers or agencies on a temporary or project-by-project basis.
1. Screen Resumes for Technical Skills
2. Look for experience in:
3. Building responsive machine learning pipelines
4 .Employing in a cloud-based environment
5. Managing manufacturing ML systems
: Technical Interview & Assessment
Add coding and system design rounds.
Check understanding of:
1.CI/CD for ML
2. Container management.
3. Monitoring & logging (e.g., Prometheus, Grafana)
4. Tracking experiments
Optional: hands-on exercise or take-home assignment (e.g., build a simple training-to-deployment pipeline).
1. Evaluate Soft Skills & Culture Fit
2. Collaboration with data scientists, software engineers, and product managers is necessary.
3. Assess communication, documentation style, and collaboration.
4. Make an Offer & Onboard
5. Offer thorough onboarding instructions.
6. Begin with a real project to see the impact soon.
Mlops engineer
???? Most Important Points to Remember MLOps ≠ DevOps: MLOps introduces additional complexity — model versioning, drift, data pipelines.
Infrastructure experience is a must: Hire individuals who have experience with cloud, containers, and orchestration tools.
Cross-function thinking: This is where MLOps intersect IT, software development, and machine learning—clear communications are crucial.
Knowledge tools: MLflow, Kubeflow, Airflow, DVC, Terraform, Docker, and Kubernetes are typical.
Security and scalability: Consider if the candidate has developed secure and scalable machine learning systems.
Model monitoring and feedback loops: Make sure they know how to check and keep the model’s performance good over time.
0 notes
Text
ARM Embedded Controllers ARMxy and Datadog for Machine Monitoring and Data Analytics
Case Details
ARM Embedded Controllers
ARM-based embedded controllers are low-power, high-performance microcontrollers or processors widely used in industrial automation, IoT, smart devices, and edge computing. Key features include:
High Efficiency: ARM architecture excels in energy efficiency, ideal for real-time data processing and complex computations.
Real-Time Performance: Supports Real-Time Operating Systems (RTOS) for low-latency industrial control.
Low Power Consumption: Optimized for continuous operation in sensors and monitoring nodes.
Flexibility: Compatible with industrial protocols (CAN, Modbus, MT connect).
Scalability: Cortex-M series for basic tasks to Cortex-A series for advanced edge computing.
Datadog
Datadog is a leading cloud-native monitoring and analytics platform for infrastructure, application performance, and log management. Core capabilities:
Data Aggregation: Collects metrics, logs, and traces from servers, cloud services, and IoT devices.
Custom Dashboards: Real-time visualization of trends and anomalies.
Smart Alerts: ML-driven anomaly detection and threshold-based notifications.
Integration Ecosystem: 600+ pre-built integrations (AWS, Kubernetes, etc.).
Predictive Analytics: Identifies patterns to forecast failures or bottlenecks.
Benefits of Combining ARM Controllers with Datadog
1. End-to-End Machine Monitoring Solution
Edge Data Collection: ARM controllers act as edge nodes, interfacing directly with sensors (e.g., temperature, vibration, current sensors).
Cloud-Based Intelligence: Data sent via MQTT/HTTP to Datadog for AI/ML-driven analysis (e.g., detecting abnormal vibration frequencies).
Use Case: Predictive maintenance for factory CNC machines by correlating sensor data with operational logs.
2. Low Latency and Real-Time Response
Edge Preprocessing: ARM controllers perform local computations (e.g., FFT analysis), reducing bandwidth usage by uploading only critical data.
Instant Alerts: Datadog triggers alerts via Slack/email for threshold breaches (e.g., overheating), minimizing downtime.
3. Remote Monitoring and Centralized Management
Global Device Oversight: Monitor distributed ARM devices worldwide via Datadog’s unified dashboard.
OTA Updates: Deploy firmware updates remotely using Datadog APIs, reducing on-site maintenance.
4. Cost and Energy Efficiency
Bandwidth Optimization: Edge computing reduces cloud storage and transmission costs.
Power-Saving Design: ARM’s low power consumption aligns with Datadog’s pay-as-you-go model for scalable deployments.
5. Scalability and Ecosystem Compatibility
Industrial Protocol Support: ARM controllers integrate with Modbus, OPC UA; Datadog ingests data via plugins or custom APIs.
Elastic Scalability: Datadog handles data from single devices to thousands of nodes without architectural overhauls.
6. Data-Driven Predictive Maintenance
Historical Insights: Datadog stores long-term data to train models predicting equipment lifespan (e.g., bearing wear trends).
Root Cause Analysis: Combine ARM controller logs with metrics to diagnose issues (e.g., power fluctuations causing downtime).
Typical Applications
Industry 4.0 Production Lines: Monitor CNC machine health with Datadog optimizing production schedules.
Wind Turbine Monitoring: ARM nodes collect gearbox vibration data; Datadog predicts failures to schedule maintenance.
Smart Buildings: ARM-based sensor networks track HVAC performance, with Datadog adjusting energy usage for sustainability.
Conclusion
The integration of ARM embedded controllers and Datadog delivers a robust machine monitoring framework, combining edge reliability with cloud-powered intelligence. The ARMxy BL410 series is equipped with 1 Tops NPU, low-power data acquisition, while Datadog enables predictive analytics and global scalability. This synergy is ideal for industrial automation, energy management, and smart manufacturing, driving efficiency and reducing operational risks.
0 notes
Text
Comparison of Ubuntu, Debian, and Yocto for IIoT and Edge Computing
In industrial IoT (IIoT) and edge computing scenarios, Ubuntu, Debian, and Yocto Project each have unique advantages. Below is a detailed comparison and recommendations for these three systems:
1. Ubuntu (ARM)
Advantages
Ready-to-use: Provides official ARM images (e.g., Ubuntu Server 22.04 LTS) supporting hardware like Raspberry Pi and NVIDIA Jetson, requiring no complex configuration.
Cloud-native support: Built-in tools like MicroK8s, Docker, and Kubernetes, ideal for edge-cloud collaboration.
Long-term support (LTS): 5 years of security updates, meeting industrial stability requirements.
Rich software ecosystem: Access to AI/ML tools (e.g., TensorFlow Lite) and databases (e.g., PostgreSQL ARM-optimized) via APT and Snap Store.
Use Cases
Rapid prototyping: Quick deployment of Python/Node.js applications on edge gateways.
AI edge inference: Running computer vision models (e.g., ROS 2 + Ubuntu) on Jetson devices.
Lightweight K8s clusters: Edge nodes managed by MicroK8s.
Limitations
Higher resource usage (minimum ~512MB RAM), unsuitable for ultra-low-power devices.
2. Debian (ARM)
Advantages
Exceptional stability: Packages undergo rigorous testing, ideal for 24/7 industrial operation.
Lightweight: Minimal installation requires only 128MB RAM; GUI-free versions available.
Long-term support: Up to 10+ years of security updates via Debian LTS (with commercial support).
Hardware compatibility: Supports older or niche ARM chips (e.g., TI Sitara series).
Use Cases
Industrial controllers: PLCs, HMIs, and other devices requiring deterministic responses.
Network edge devices: Firewalls, protocol gateways (e.g., Modbus-to-MQTT).
Critical systems (medical/transport): Compliance with IEC 62304/DO-178C certifications.
Limitations
Older software versions (e.g., default GCC version); newer features require backports.
3. Yocto Project
Advantages
Full customization: Tailor everything from kernel to user space, generating minimal images (<50MB possible).
Real-time extensions: Supports Xenomai/Preempt-RT patches for μs-level latency.
Cross-platform portability: Single recipe set adapts to multiple hardware platforms (e.g., NXP i.MX6 → i.MX8).
Security design: Built-in industrial-grade features like SELinux and dm-verity.
Use Cases
Custom industrial devices: Requires specific kernel configurations or proprietary drivers (e.g., CAN-FD bus support).
High real-time systems: Robotic motion control, CNC machines.
Resource-constrained terminals: Sensor nodes running lightweight stacks (e.g., Zephyr+FreeRTOS hybrid deployment).
Limitations
Steep learning curve (BitBake syntax required); longer development cycles.
4. Comparison Summary
5. Selection Recommendations
Choose Ubuntu ARM: For rapid deployment of edge AI applications (e.g., vision detection on Jetson) or deep integration with public clouds (e.g., AWS IoT Greengrass).
Choose Debian ARM: For mission-critical industrial equipment (e.g., substation monitoring) where stability outweighs feature novelty.
Choose Yocto Project: For custom hardware development (e.g., proprietary industrial boards) or strict real-time/safety certification (e.g., ISO 13849) requirements.
6. Hybrid Architecture Example
Smart factory edge node:
Real-time control layer: RTOS built with Yocto (controlling robotic arms)
Data processing layer: Debian running OPC UA servers
Cloud connectivity layer: Ubuntu Server managing K8s edge clusters
Combining these systems based on specific needs can maximize the efficiency of IIoT edge computing.
0 notes